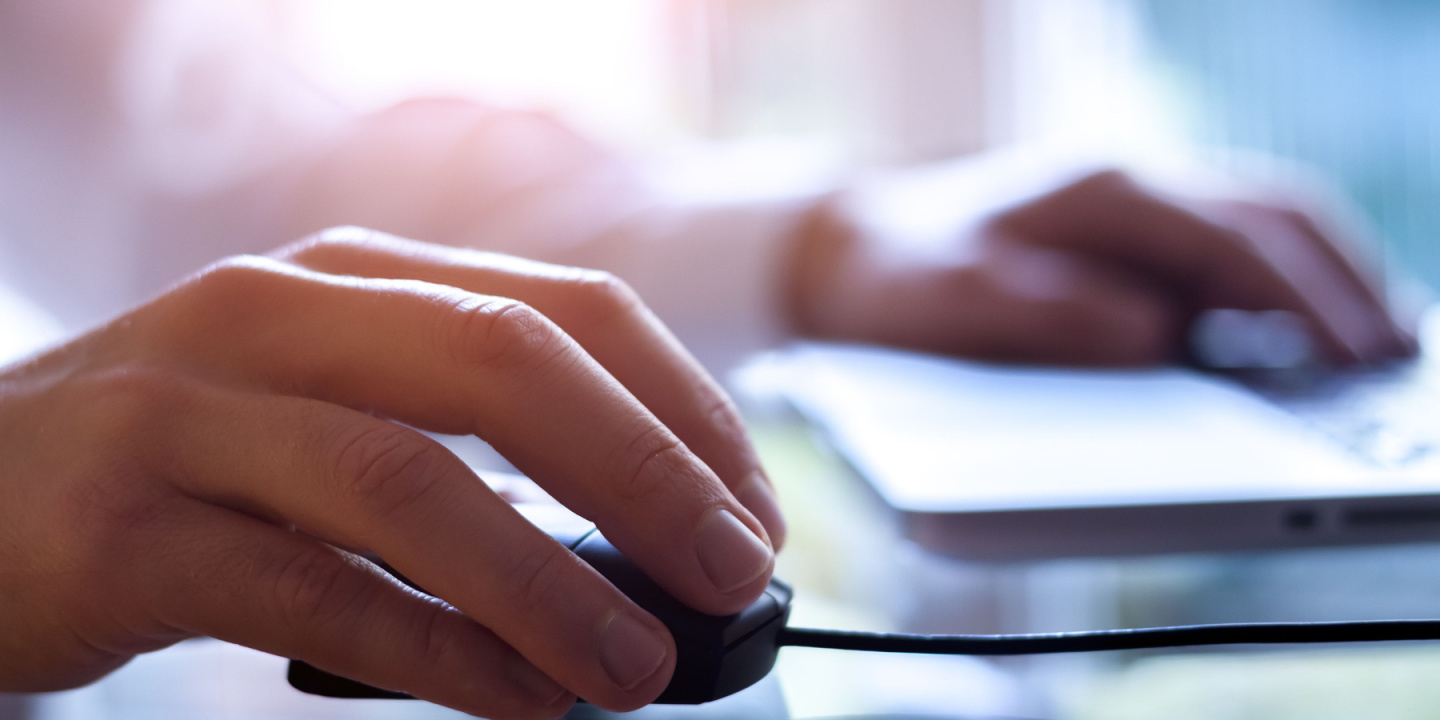
Using online assessment behaviour to identify student thinking
6 Dec 2022 5 minute readUnderstanding how a student answers online assessments could provide valuable insights for teaching and learning.
Is it possible to use data from computer-delivered assessments to understand how a reader has engaged with material? New research by the Centre for International Student Assessment in Germany and ACER suggests it is. This research shows that the identification of patterns in reader behaviour can provide valuable insights that may inform educational interventions.
Traditional paper-based assessments collect only the final product of a test-taker’s thinking. In a computer-based assessment, the data capture can give insights on the processes students followed in reaching their response.
The initial rationale for using computers in assessments was greater efficiency. If scoring and reporting could be automated, or testing made adaptive, assessments could be delivered more cheaply, feedback could be given quickly, and testing itself could be more targeted. More recently, the potential of computer-delivered assessments to give insight into these previously inaccessible processes has shown to be possibly of even greater importance.
Generally, computer-based assessments capture 3 kinds of data, collectively known as log-file, or process data. It can capture:
- the type of interaction that the student has with the material. At the most general level, this includes mouse clicks. Depending on the assessment, it might also include interactions such as mouse down/up rollover actions, dragging and dropping an item from one place on the screen to another, choosing an option from a drop-down menu or entering text
- the frequency of interaction. Knowing that a student clicked on a particular button or link gives us one kind of information. Knowing that they clicked on the same button multiple times may give us a different kind of information
- the time taken. Each individual action completed by a student has a time stamp, making it possible to examine both the total time taken to complete an item, and the time taken to complete individual steps within an item.
The study used data from the 2012 Programme for International Student Assessment (PISA), which included an online reading assessment. In various tasks, the participants had to find information, integrate it within or across texts, and reflect on and evaluate what they had read.
Based on previous work, researchers expected to find at least three patterns:
- an on-task pattern, in which student behaviour meets the requirements of respective tasks i.e. precision and processing times that reflect task demands, with less-complex tasks being processed faster than more complex ones
- an exploring pattern, in which students visit many hypertext pages and show low navigational precision and long processing times in the first item of a digital reading unit
- a disengaged pattern, in which students show generally low activity in navigation, short processing times and relatively long orientation times, potentially indicating struggle or disinterest.
On-task behaviours were observed for many PISA 2012 students. The flow across the multiple units indicated that most students, independent from their behaviour in the first unit, exhibited on-task behaviour in later units. The study indicated that students with low print-reading skills were more likely to show a disengaged pattern than proficient readers. However, it remains unclear whether students struggle to understand the texts they are reading, or the demands of the task once they have done so.
The study emphasises that both task requirements and the assessment context must be considered when interpreting log-file data. To make the best use of these activity patterns requires careful preparation during item and test development and piloting. While simple indicators provide insight into how students interact with digital reading tasks, depending on the task/context, they may not allow for unambiguous inferences at the individual level. For example, proficient readers can be expected to process simple reading tasks faster than less proficient readers. However, the processing time of students who are good readers and show the exploring pattern is substantially higher than that of students showing the exploring pattern who were poor readers. This suggests that while each indicator will provide independent information about how a student has behaved, it is also helpful to examine the pattern of behaviour revealed by the collective information from all the indicators.
This research shows that the identification of patterns in reader behaviour can provide useful insights into informing educational interventions. A suggested first-step would be to recognise the disengaged pattern. This could detect disengaged test-takers and teachers could intervene to motivate students or determine reasons for their disengagement, rather than assess them only on the final test result.
Find out more:
More information is available in the full study ‘Patterns of reading behaviour in digital hypertext environments’by Carolin Hahnel, Dara Ramalingam, Ulf Kroehne and Frank Goldhammer.