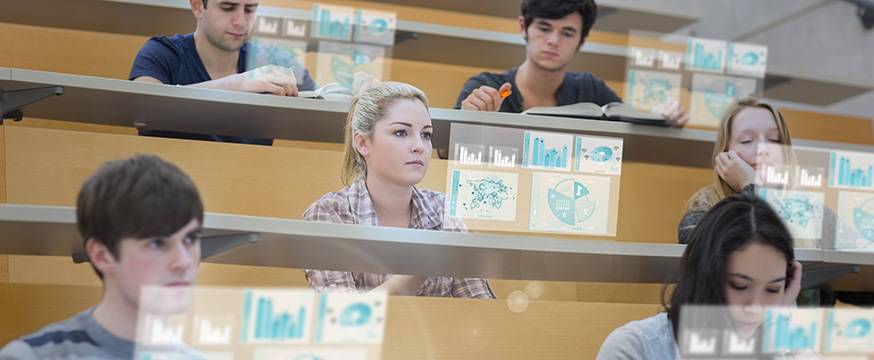
Big data in higher education
Research 12 May 2015 6 minute readMichael Timms explains how the emerging field of learning analytics is helping tertiary institutions identify and address the individual needs of students.
Big data in higher education
As more students study courses delivered totally or partially online, the disconnect between students and universities is making it harder to keep a finger on the pulse, to check how individual students are faring.
At the same time, many institutions are gathering increasing amounts of data on students and are keen to gain more useful information from this to support students’ individual needs. The higher education sector has spearheaded the move of education into the era of big data and learning analytics.
Big data
There are many sources of data and information about students.
In addition to information on student academic achievement, institutions have automated systems that track student timetables, record absences and provide other information that might be relevant to how students are performing. There are also sources of additional data on students’ background, including eligibility for special services, proficiency in the language of instruction, disability, age and gender.
Increasingly, institutions are looking to combine these data sources in ways that will help them to support their students in a more personalised and evidence-based way.
In the field of education, two strands of research have developed which are both concerned with the analysis and interpretation of big data – educational data mining and learning analytics.
Educational data mining reduces learning into small components that can be analysed in order to look for new patterns in data. These patterns can then be used to develop new algorithms that enable educational software to adapt to a student.
Learning analytics is the process of developing actionable insights through problem definition and the application of statistical models and analysis against existing data or even simulated future data.
Predictive modelling with learning analytics
What makes learning analytics different from traditional data analysis is that it uses sophisticated techniques to build predictive models in instructional systems. Predictive models are derived from analyses of existing data that are predictive of certain outcomes.
Once a predictive model has been created based on existing data, it can be tested on a different batch of existing data to check how reliable it is. Having been established as reliable, the model is then ready to be applied to predict from current data.
The advantage of using predictive models is that they provide early warnings before an undesirable event happens, so that interventions can take place to stop it happening.
Learning analytics in practice
There has been a lot of work in higher education on the development of models that predict student dropout. Through analysis of the existing data a quantitative model can be built that provides an estimate of the probability that a student might drop out, based on a combination of other variables.
A model that predicts the likelihood of a student dropping out might take inputs from a Learning Management System to monitor things like whether a student has attended class, handed in work on time, participated in online discussions, downloaded learning materials and so on. Then the model can estimate, in real time, if a student is at risk of dropping out.
The University of Alabama in the United States has applied learning analytics to improve student retention between the first and second years of study. They built a predictive model that used data on students’ average grades, grades in maths and English courses, distance travelled to campus, race, total hours and highest score on university entrance exams. This retention model is used each year with other data to identify 150-200 first-year students who are not likely to return for their second year. The information is then shared with faculty and academic advisors for outreach efforts to prevent their likely dropout.
Also in the US, Northern Arizona University has developed a predictive model that helps to identify students who need to use its academic and other support resources before they are at serious risk of failure or withdrawal. Under the old system, resources were usually only used when individual students were in real trouble and the chance of rescuing them before they failed was low. Data have shown that when students use the five different types of support services, their academic performance is enhanced.
Challenges ahead
While the possibilities of big data and learning analytics are exciting, there are many challenges ahead.
On the technical side, there are challenges to integrate data from different sources, on different platforms and from different vendors that were not designed to work with one another. This will requires the development of standards of interoperability so that data are tagged in consistent ways across systems, as well as software solutions to pull those data together.
On the practical side, institutions and their staff will have to develop new levels of expertise in data management and analysis.
On the political side, as more and more data become available and are manipulated in different ways, issues of privacy and data protection become important. Institutions and education systems will have to navigate their way through these concerns to develop procedures that allow them to access and process personal data for educational purposes, while ensuring that they collect data in sensitive ways, protect those data and ensure that they are used only by authorised users for agreed purposes.
Further information:
This article is based on a Centre for Strategic Education occasional paper, ‘Big Data in Education: A guide for educators’, by Dr Michael Timms.